ALL-E: Aesthetics-guided Low-light Image Enhancement
Abstract
Evaluating the performance of low-light image enhancement (LLE) is highly subjective, thus making integrating human preferences into image enhancement a necessity. Existing methods fail to consider this and present a series of potentially valid heuristic criteria for training enhancement models. In this paper, we propose a new paradigm,i.e., aesthetics-guided low-light image enhancement (ALL-E), which introduces aesthetic preferences to LLE and motivates training in a reinforcement learning framework with an aesthetic reward. Each pixel, functioning as an agent, refines itself by recursive actions, i.e., its corresponding adjustment curve is estimated sequentially. Extensive experiments show that integrating aesthetic assessment improves both subjective experience and objective evaluation. Our results on various benchmarks demonstrate the superiority of ALL-E over state-of-the-art methods. Source code and models are in the supplementary material for evaluation.
Method
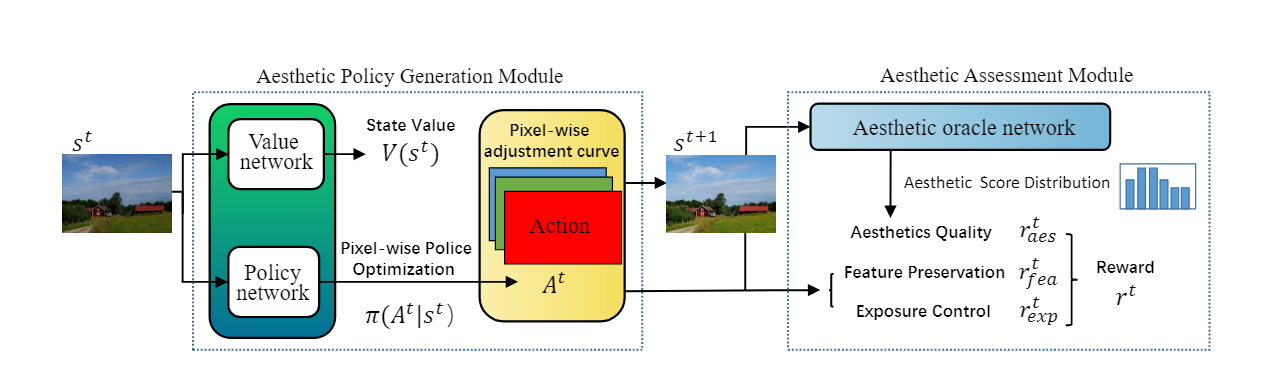
Overall architecture of our proposed ALL-E. We devise aesthetics-guided LLE (ALL-E) through reinforcement learning and treat LLE as a Markov decision process, which divides the LLE process into two phases: aesthetic policy generation and aesthetic assessment.
Contributions
We propose a new paradigm for LLE by integrating aesthetic assessment, leveraging aesthetic scores to mimic human subjective evaluations as a reward to guide LLE. To the best of our knowledge, this is the first attempt to solve low-light image enhancement using aesthetics
We devise aesthetics-guided LLE (ALL-E) through reinforcement learning and treat LLE as a Markov decision process, which divides the LLE process into two phases: aesthetic policy generation and aesthetic assessment.
Our method is evaluated against state-of-the-art competitors through comprehensive experiments in terms of visual quality, no- and full-referenced image quality assessment, and human subjective surveys. All results consistently demonstrate the superiority of ALL-E.
Results
Visual quality comparison
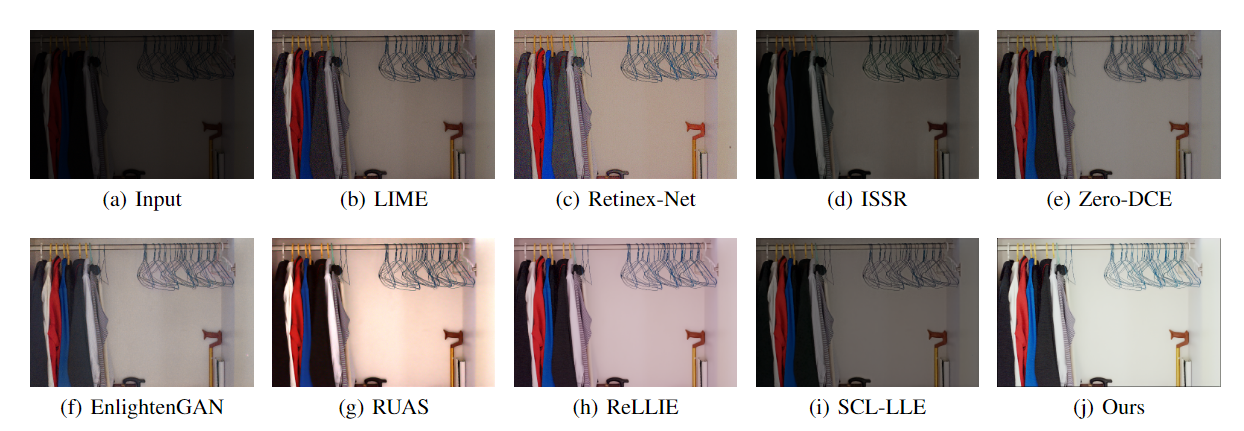
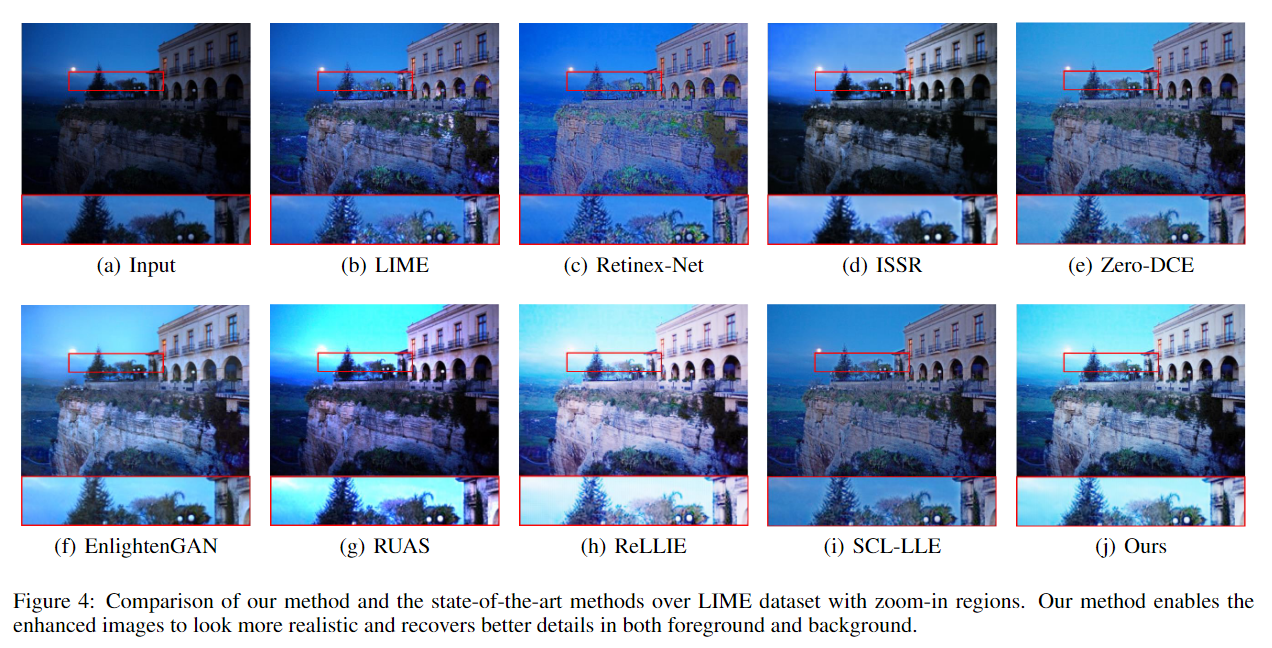
Image quality assessment
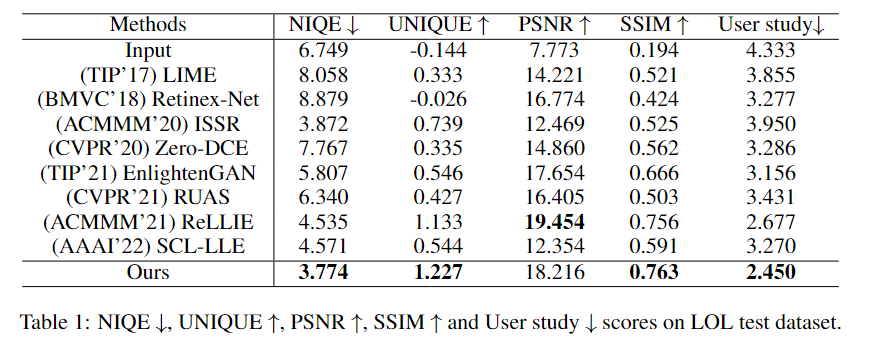
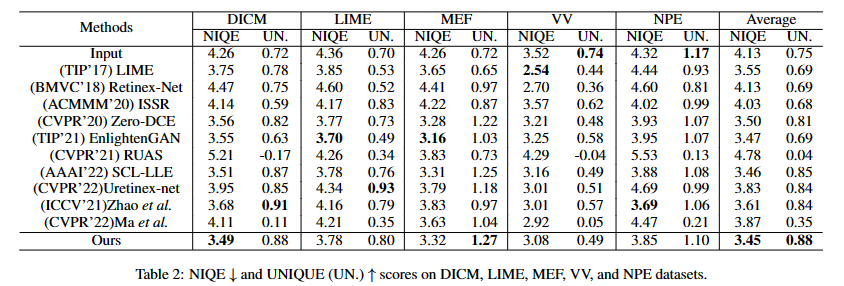
Ablation Studies
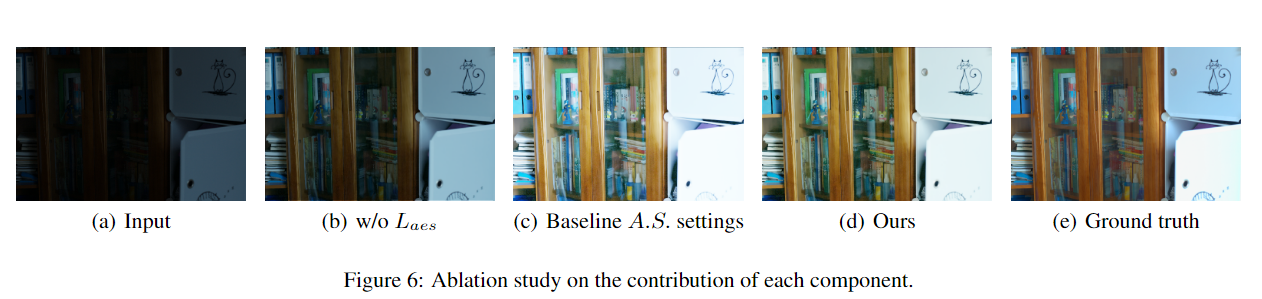
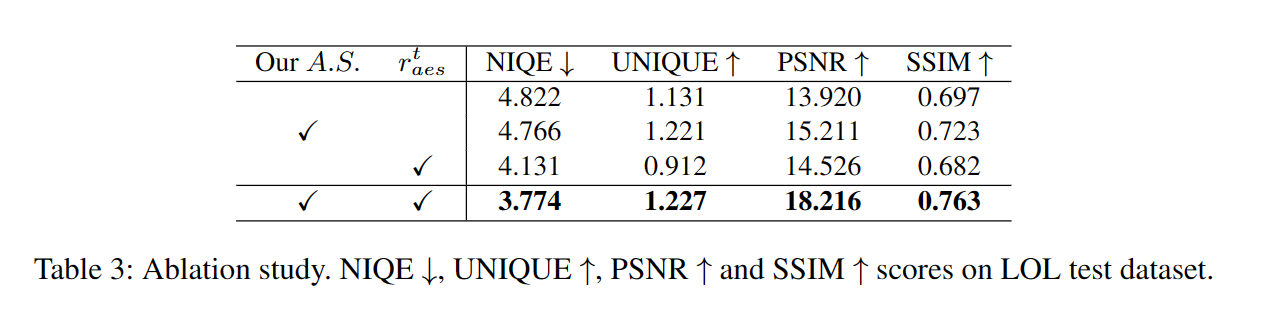
Citation
@article{li2023alle, title = {ALL-E: Aesthetics-guided Low-light Image Enhancement}, author ={Ling Li and Dong Liang and Yuanhang Gao and Sheng-Jun Huang and Songcan Chen}, booktitle = {Proceedings of the Thirty-Second International Joint Conference on Artificial Intelligence, {IJCAI-23}}, publisher = {International Joint Conferences on Artificial Intelligence Organization}, year={2023}, month = {8}, pages = {1062--1070} }
Contact
If you have any questions, please contact Dong Liang at liangdong@nuaa.edu.cn or gaoyuanhang@nuaa.edu.cn.