Anchor Retouching via Model Interaction for Robust
Object Detection in Aerial Images
Abstract
Object detection has made tremendous strides in computer vision. Small object detection with appearance degradation is a prominent challenge, especially for aerial observations. To collect sufficient positive/negative samples for heuristic training, most object detectors preset region anchors in order to calculate intersection-over-union (IoU) against the ground-truth data. In this case, small objects are frequently abandoned or mislabeled. In this article, we present an effective dynamic enhancement anchor network (DEA-Net) to construct a novel training sample generator. Different from the other state-of-the-art (SOTA) techniques, the proposed network leverages a sample discriminator to realize interactive sample screening between an anchor-based unit and an anchor-free unit to generate eligible samples. Besides, multi-task joint training with a conservative anchor-based inference scheme enhances the performance of the proposed model while reducing computational complexity. The proposed scheme supports both oriented and horizontal object detection tasks. Extensive experiments on two challenging aerial benchmarks (i.e., Dataset of Object deTection in Aerial images (DOTA) and HRSC2016) indicate that our method achieves SOTA performance in accuracy with moderate inference speed and computational overhead for training. On DOTA, our DEA-Net which integrated with the baseline of RoI-transformer surpasses the advanced method by 0.40% mean-average-precision (mAP) for oriented object detection with a weaker backbone network (ResNet-101 vs. ResNet-152) and 3.08% mAP for horizontal object detection with the same backbone. Besides, our DEA-Net which integrated with the baseline of ReDet achieves the SOTA performance by 80.37%. On HRSC2016, it surpasses the previous best model by 1.1% using only three horizontal anchors. The source code and the training set are made publicly available at https://github.com/QxGeng/DEA-Net..
Method
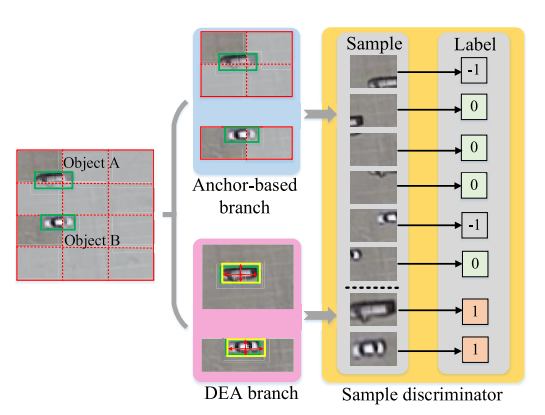
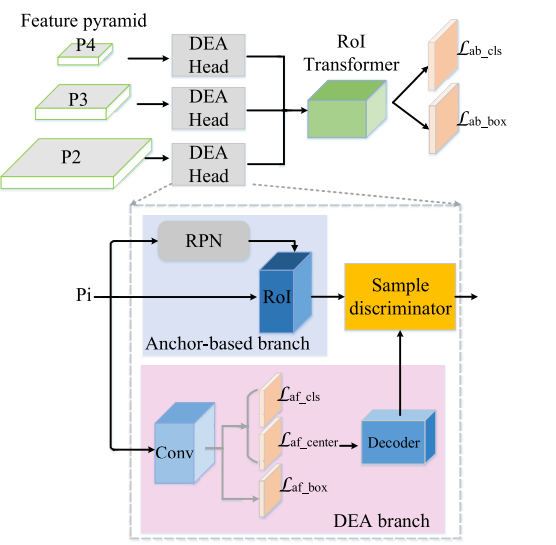
DEA module to provide better positive samples of small objects.(left)
Architecture of our proposed DEA-Net for oriented object detection.(right)
Contributions
an effective sample generator based on DEA head to enhance the performance of detecting small objects by combining the advantages of anchor-based and anchor-free model.
a novel and robust DEA-Net, which can achieve the start-of-the-art oriented and horizontal object detection performance in aerial images.
Results
IoU distributions of the baseline (faster R-CNN) and our DEAnet
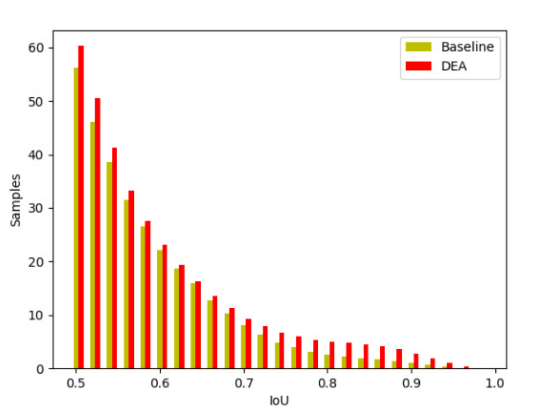
The x-axis represents the IoU between the samples and the ground-truth boxes. The y-axis represents the average number of samples on each image.
Visualization results
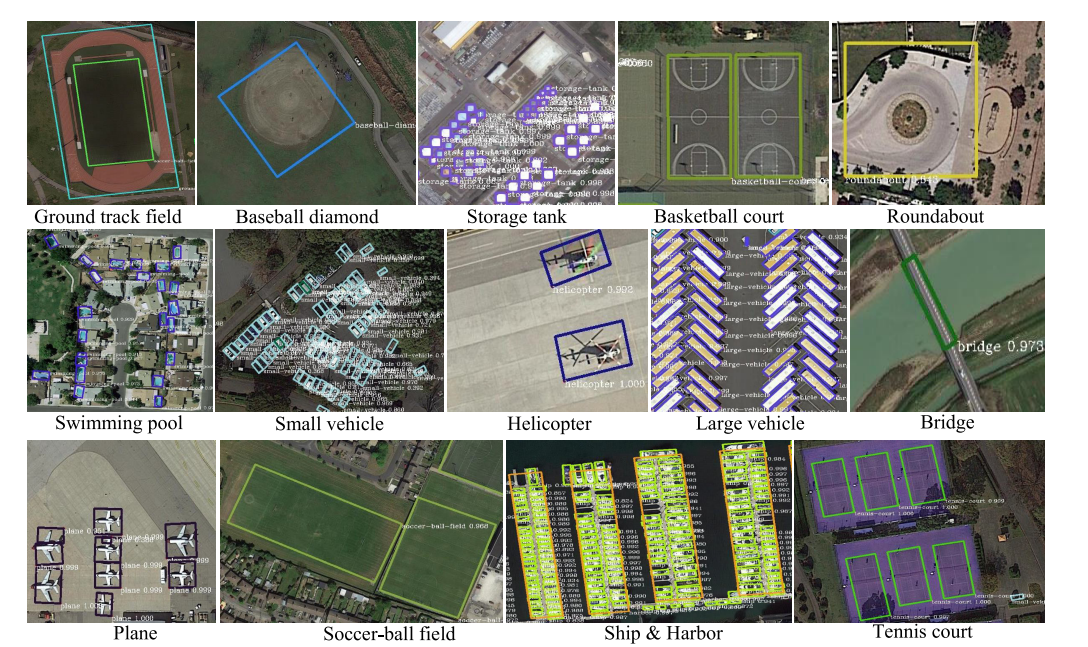
Comparisons With State-of-the-Arts
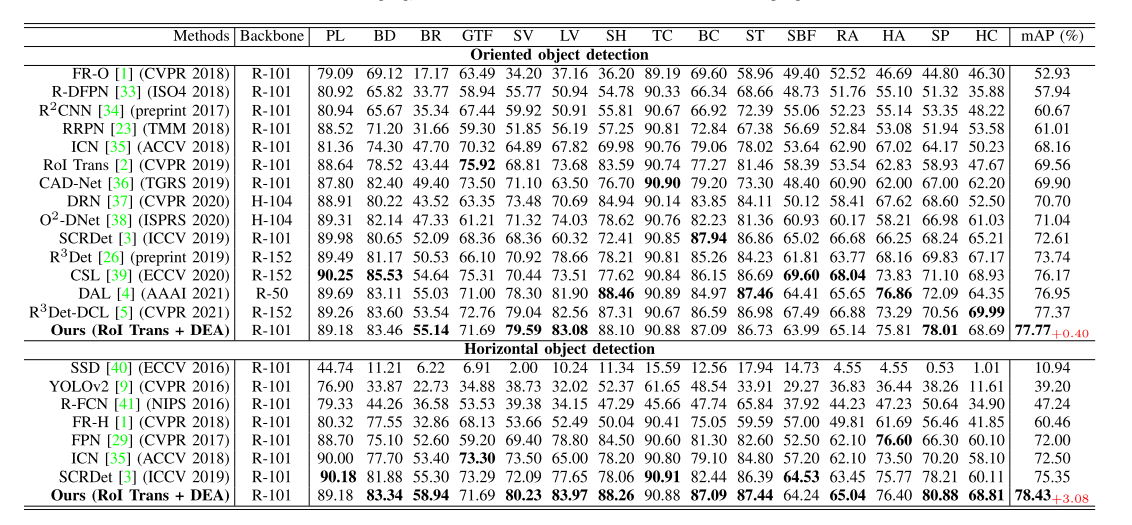

Ablation Studies
Effectiveness and Efficiency on Different Backbones & Effectiveness of the Proposed Units

Efficiency of Our Proposed Units

Effectiveness of the Inference Schemes
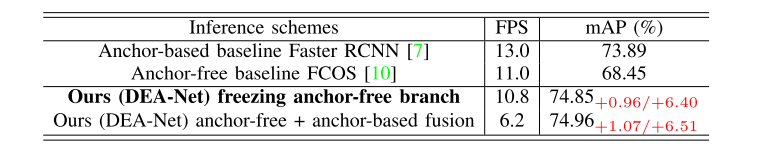
Citation
@ARTICLE{9654160, author={Liang, Dong and Geng, Qixiang and Wei, Zongqi and Vorontsov, Dmitry A. and Kim, Ekaterina L. and Wei, Mingqiang and Zhou, Huiyu}, journal={IEEE Transactions on Geoscience and Remote Sensing}, title={Anchor Retouching via Model Interaction for Robust Object Detection in Aerial Images}, year={2022}, volume={60}, number={}, pages={1-13}, doi={10.1109/TGRS.2021.3136350}}
Contact
If you have any questions, please contact Dong Liang at liangdong@nuaa.edu.cn.