Semantically Contrastive Learning for
Low-Light Image Enhancement
2 Nanjing University of Aeronautics and Astronautics Shenzhen Research Institute, Nanjing, China
Abstract
In this paper, we propose a physics-inspired contrastive learning paradigm for low-light enhancement, called PIE. PIE primarily addresses three issues: (i) To resolve the problem of existing learning-based methods often training a LLE model with strict pixel-correspondence image pairs, we eliminate the need for pixel-correspondence paired training data and instead train with unpaired images. (ii) To address the disregard for negative samples and the inadequacy of their generation in existing methods, we incorporate physics-inspired contrastive learning for LLE and design the Bag of Curves (BoC) method to generate more reasonable negative samples that closely adhere to the underlying physical imaging principle. (iii) To overcome the reliance on semantic ground truths in existing methods, we propose an unsupervised regional segmentation module, ensuring regional brightness consistency while eliminating the dependency on semantic ground truths. Overall, the proposed PIE can effectively learn from unpaired positive/negative samples and smoothly realize non-semantic regional enhancement, which is clearly different from existing LLE efforts. Besides the novel architecture of PIE, we explore the gain of PIE on downstream tasks such as semantic segmentation and face detection. Training on readily available open data and extensive experiments demonstrate that our method surpasses the state-of-the-art LLE models over six independent cross-scenes datasets. PIE runs fast with reasonable GFLOPs in test time, making it easy to use on mobile devices.
Method
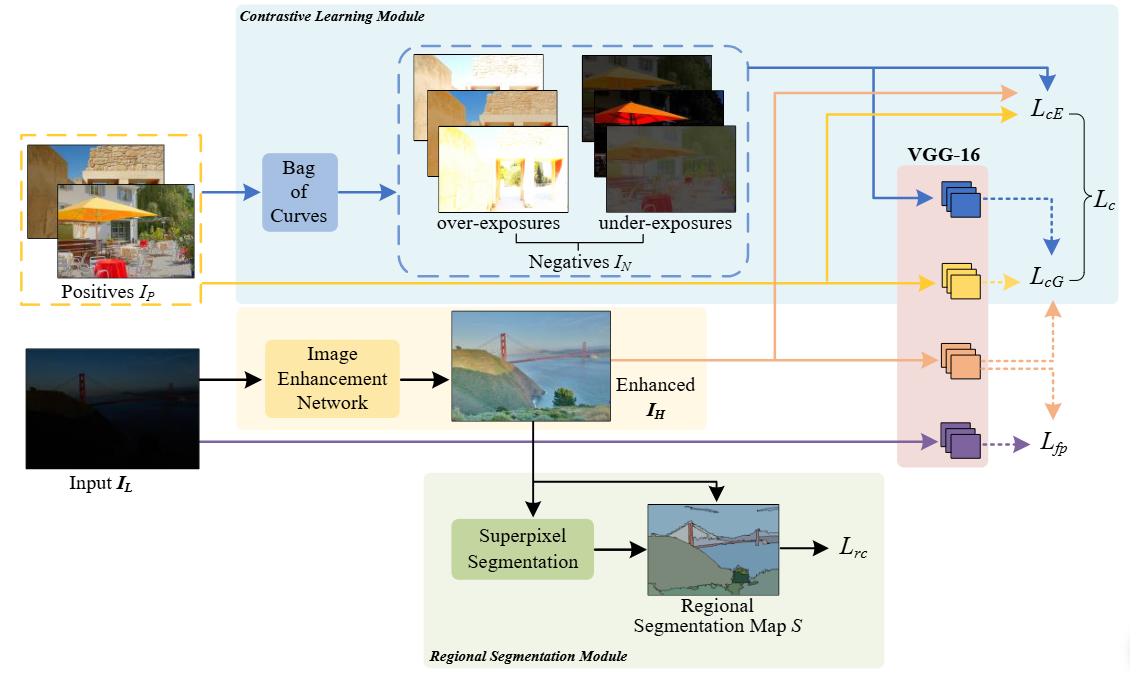
The overall architecture of PIE. It includes a low-light image enhancement (LLE) network, a contrastive learning module (the blue block) boosted by Bag of Curves (BoC), a regional segmentation module (the green block), and a VGG-16 feature extractor (the red block).
Contributions
A physics-inspired contrastive learning approach for real-world cross-scene LLE, without any paired training images and pixel-level annotation:
1) a physics-inspired approach called “Bag of Curves" generates negative samples for contrastive learning using principles closer to the underlying physical imaging mechanism.
2) an unsupervised regional segmentation module to maintain regional brightness consistency, realize region-discriminate enhancement, and release from semantic labels.
3) a multi-task joint learning with three constraints -- contrastive learning, regional brightness consistency, and feature preservation, simultaneously ensuring exposure, texture, and color consistency.PIE is compared with SOTAs via comprehensive experiments on six independent datasets in terms of visual quality, no and full-referenced image quality assessment, and human subjective survey. All results consistently endorse the superiority and efficiency of the proposed approach.
We demonstrate that our PIE is friendly to downstream high-level vision tasks and easy to joint-learn with them.
Results
Visual quality comparison
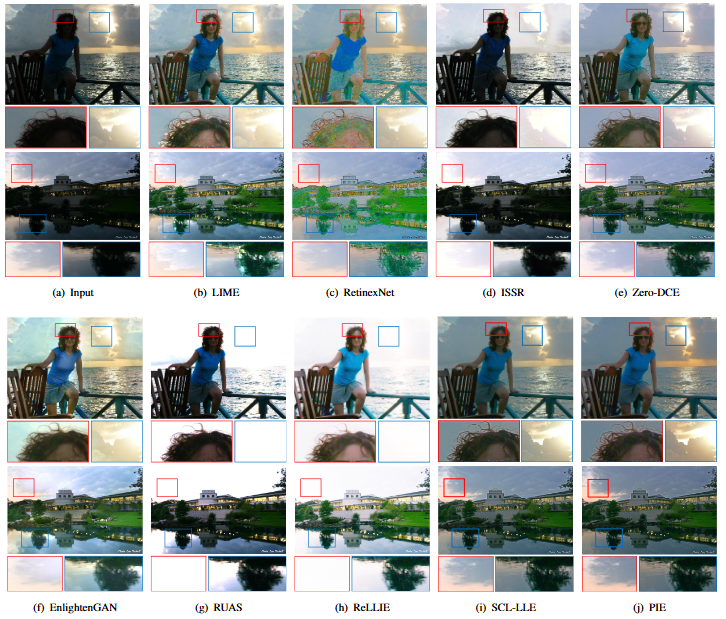
No-referenced image quality assessment
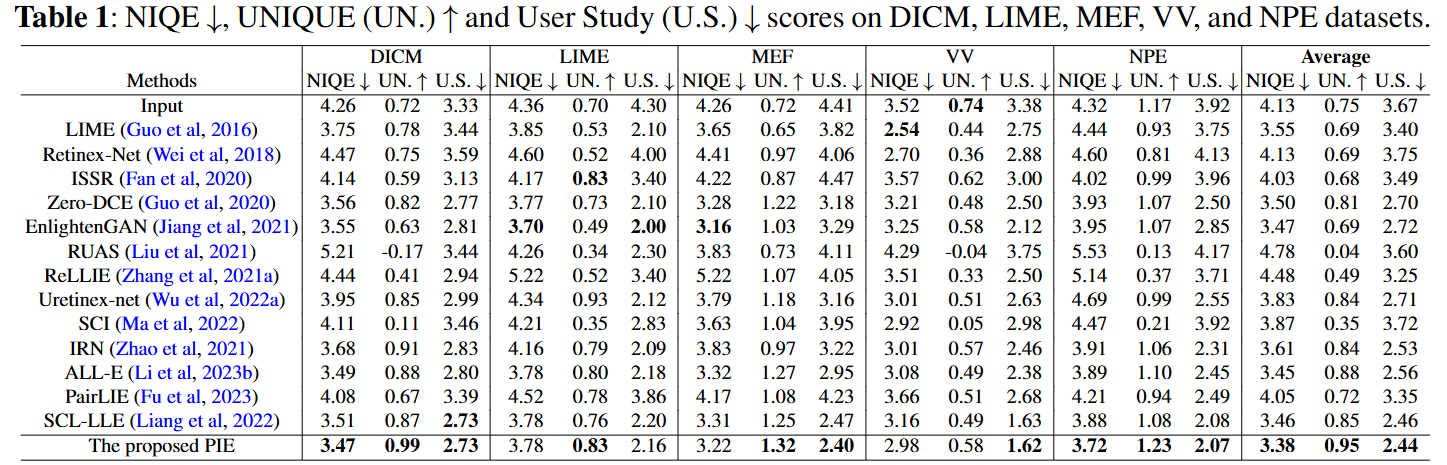
Full-referenced image quality assessment

Ablation Studies
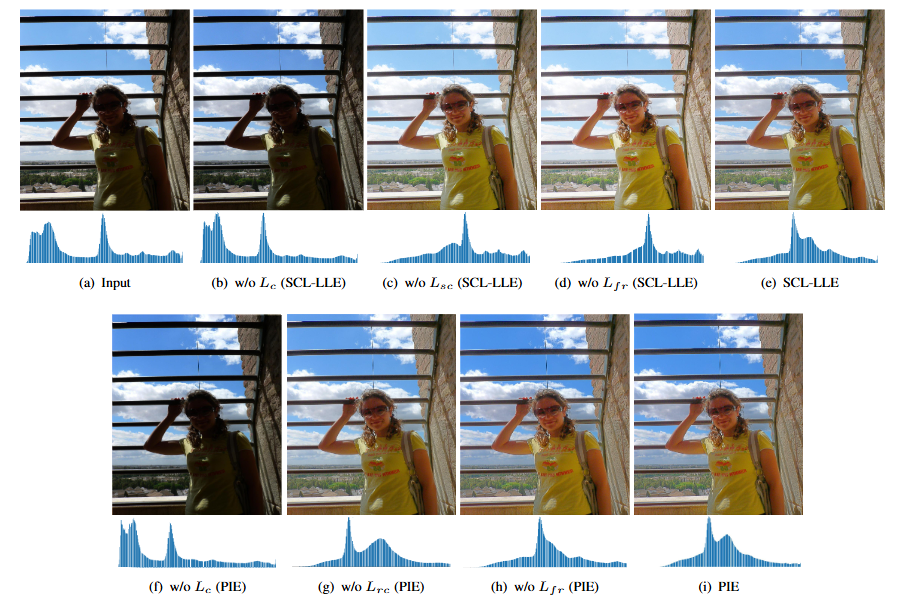
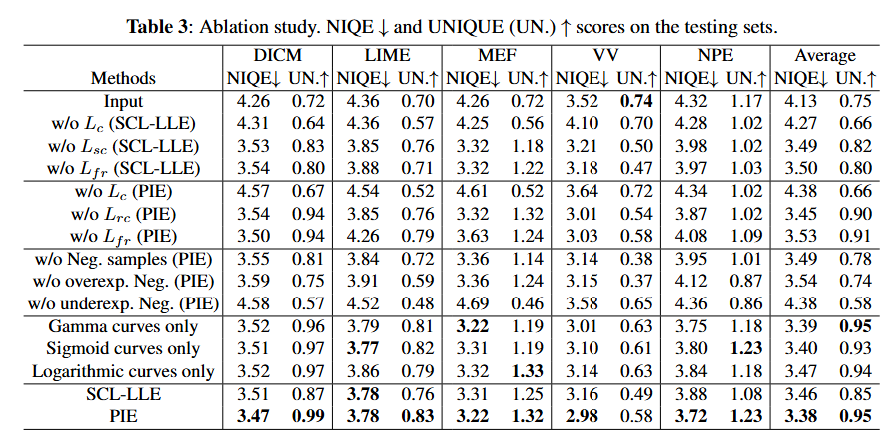
Citation
@article{liang2024pie, title={PIE: Physics-Inspired Low-Light Enhancement}, author={Liang, Dong and Xu, Zhengyan and Li, Ling and Wei, Mingqiang and Chen, Songcan}, journal={International Journal of Computer Vision}, pages={1--22}, year={2024}, publisher={Springer} }
Contact
If you have any questions, please contact Dong Liang at liangdong@nuaa.edu.cn.