Semantically Contrastive Learning for
Low-Light Image Enhancement
2 vivo Mobile Communication
3 Nanyang Technological University
4 University of Leicester
Abstract
Low-light image enhancement (LLE) remains challenging due to the unfavorable prevailing low-contrast and weak-visibility problems of single RGB images. In this paper, we respond to the intriguing learning-related question – if leveraging both accessible unpaired over/underexposed images and high-level semantic guidance, can improve the performance of cutting-edge LLE models? Here, we propose an effective semantically contrastive learning paradigm for LLE (namely SCL-LLE). Beyond the existing LLE wisdom, it casts the image enhancement task as multi-task joint learning, where LLE is converted into three constraints of contrastive learning, semantic brightness consistency, and feature preservation for simultaneously ensuring the exposure, texture, and color consistency. SCL-LLE allows the LLE model to learn from unpaired positives (normal-light)/negatives (over/underexposed), and enables it to interact with the scene semantics to regularize the image enhancement network, yet the interaction of high-level semantic knowledge and the lowlevel signal prior is seldom investigated in previous methods. Training on readily available open data, extensive experiments demonstrate that our method surpasses the state-of-thearts LLE models over six independent cross-scenes datasets. Moreover, SCL-LLE's potential to benefit the downstream semantic segmentation under extremely dark conditions is discussed. Source Code: https://github.com/LingLIx/SCL-LLE.
Method
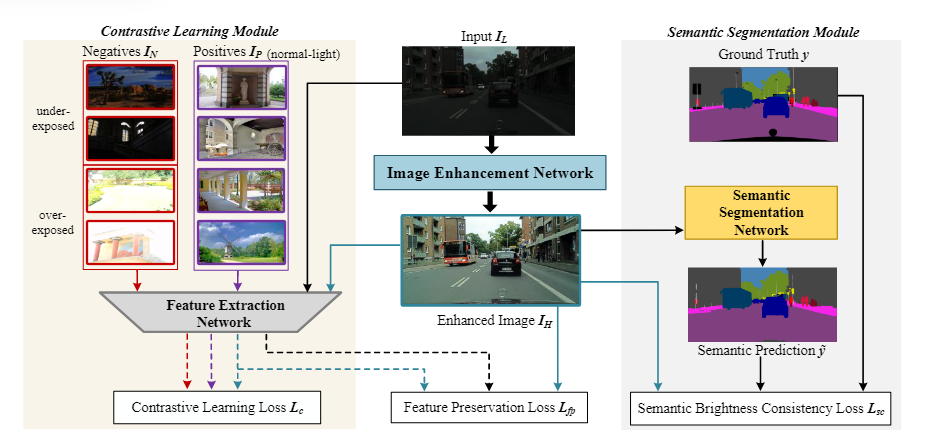
Overall architecture of our proposed SCL-LLE. It includes a low-light image enhancement network, a contrastive learning module and a semantic segmentation module
Contributions
SCL-LLE removes pixel-correspond paired training data, and provides a more flexible way:
1) training with unpaired images in different real-world domains
2) training with unpaired negative samples, allowing us to leverage readily available open data to build a more generalized and discriminative LLE networkLow and high-level vision tasks (i.e., LLE and semantic segmentation) promote each other. A semantic brightness consistency loss is introduced to ensure smooth and natural brightness recovery of the same semantic category. The enhanced images lead to better performance on the downstream semantic segmentation.
SCL-LLE is compared with SOTAs via comprehensive experiments on six independent datasets, in terms of visual quality, no and full-referenced image quality assessment, and human subjective survey. All results consistently endorse the superiority of the proposed approach.
Results
Visual quality comparison
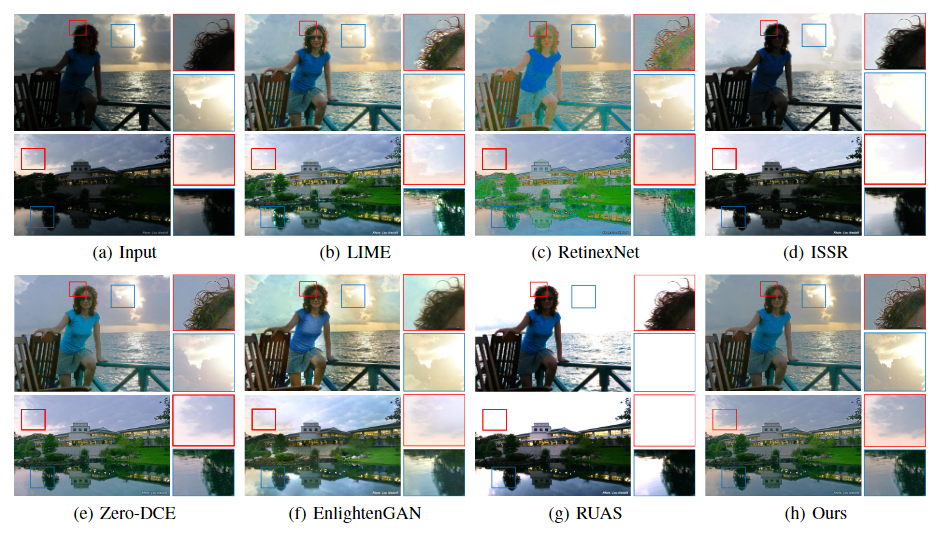
No-referenced image quality assessment
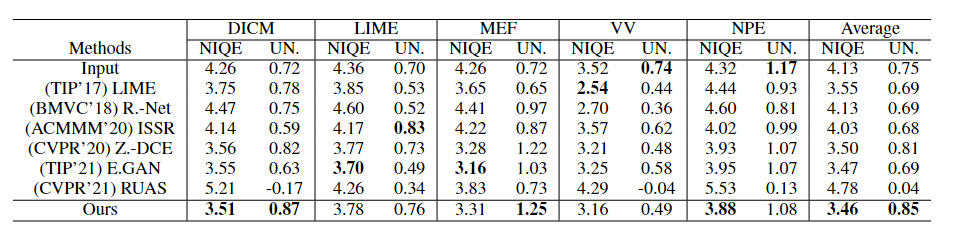
Full-referenced image quality assessment

Ablation Studies
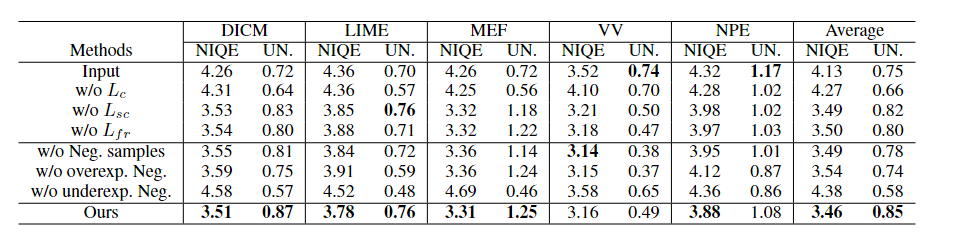
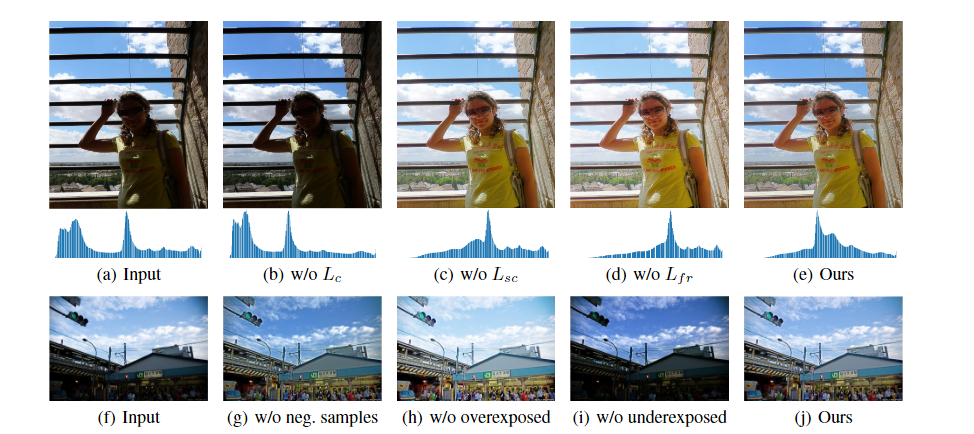
Citation
@inproceedings{liang2022semantically, title={Semantically contrastive learning for low-light image enhancement}, author={Liang, Dong and Li, Ling and Wei, Mingqiang and Yang, Shuo and Zhang, Liyan and Yang, Wenhan and Du, Yun and Zhou, Huiyu}, booktitle={Proceedings of the AAAI Conference on Artificial Intelligence}, volume={36}, number={2}, pages={1555--1563}, year={2022}}
Contact
If you have any questions, please contact Dong Liang at liangdong@nuaa.edu.cn.