Aesthetics-guided Low-light Enhancement
Abstract
Evaluating the performance of low-light image enhancement (LLE) is highly subjective, thus making integrating human preferences into LLE a necessity. Existing methods fail to consider this and present a series of potentially valid heuristic criteria for training LLE models. In this paper, we propose a new paradigm, i.e., aesthetics-guided low-light image enhancement (ALL-E), which introduces aesthetic preferences to LLE and motivates training in a reinforcement learning framework with an aesthetic reward. Each pixel, functioning as an agent, refines itself by recursive actions. We further present ALL-E+, an extended version of ALL-E, which casts a two-stage aesthetics-guided enhancement and denoising. ALL-E+ achieves low-light enhancement and denoising compensation sequentially in a unified framework, resulting in significant improvements in both subjective visual experience and objective evaluation. Extensive experiments show that integrating aesthetic preferences can further improve the visual experience of enhanced images. Our results on various benchmarks also demonstrate the superiority of our method over state-of-the-art methods. Source code is available at: https://dongl-group.github.io/project_pages/ALLE+.html
Method
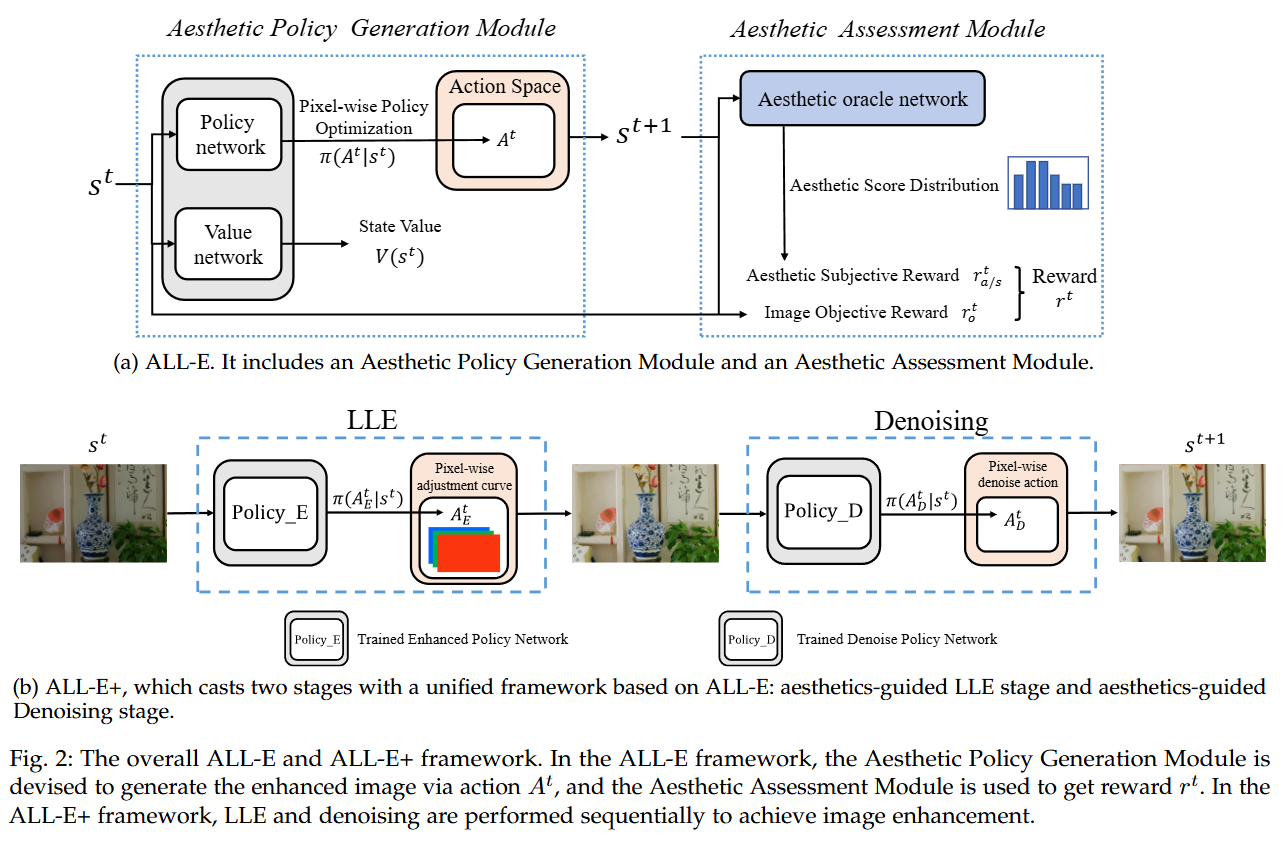
The overall ALL-E and ALL-E+ framework. In the ALL-E framework, the Aesthetic Policy Generation Module is devised to generate the enhanced image via action At, and the Aesthetic Assessment Module is used to get reward rt. In the ALL-E+ framework, LLE enhancement and denoising are performed sequentially to achieve image enhancement.
This work is an extension of our earlier work ALL-E that appeared at IJCAI-23. We have expanded and introduced many new parts compared to the conference version. First, we proposed ALL-E+, an extended version of ALL-E equipped with denoising capabilities to remove the boosted noise effectively. Second, we have improved the aesthetic quality reward and divided the reward into aesthetic subjective reward and image objective reward to ensure better subjective visual experience and objective evaluation. Third, we conducted experiments based on different aesthetic oracle networks to discuss the impact of the networks further.
Contributions
A new paradigm for LLE integrating image aesthetics assessment, leveraging aesthetic score to mimic human subjective assessment as a reward to guide the enhancement. To our knowledge, this is the first attempt to solve LLE using aesthetics. We devise aesthetics-guided LLE (ALL-E) through reinforcement learning and treat LLE as a Markov decision process, which divides LLE into two phases: aesthetic policy generation and aesthetic assessment.
We propose ALL-E+, which casts the low-light image enhancement into two stages - enhancement and denoising, leveraging the unified reinforcement learning framework. ALL-E+ further improves ALL-E by incorporating noise removal capability, thereby enabling comprehensive and effective enhancement.
ALL-E+ is evaluated against state-of-the-art competitors through comprehensive experiments in terms of visual quality, no- and full-referenced image quality assessment, and human subjective survey. All results consistently demonstrate the superiority of ALL-E+.
Results
Image quality assessment
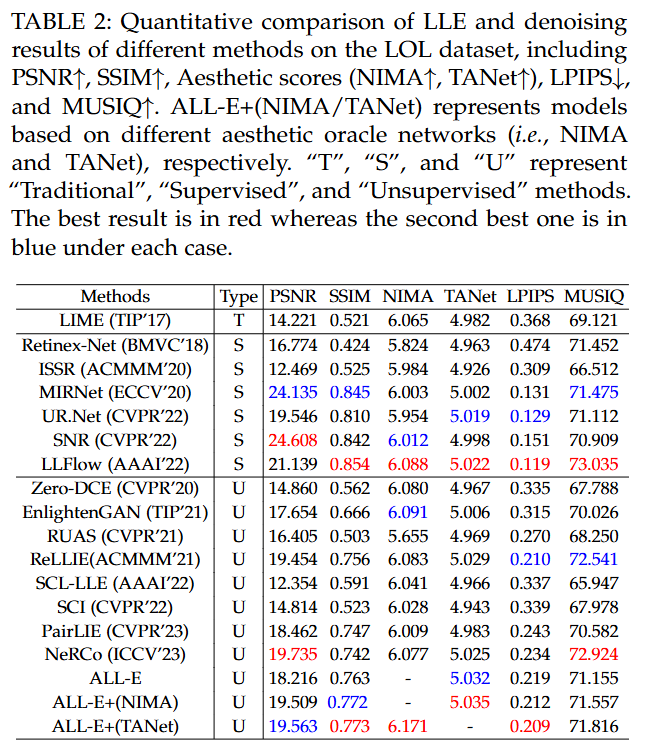
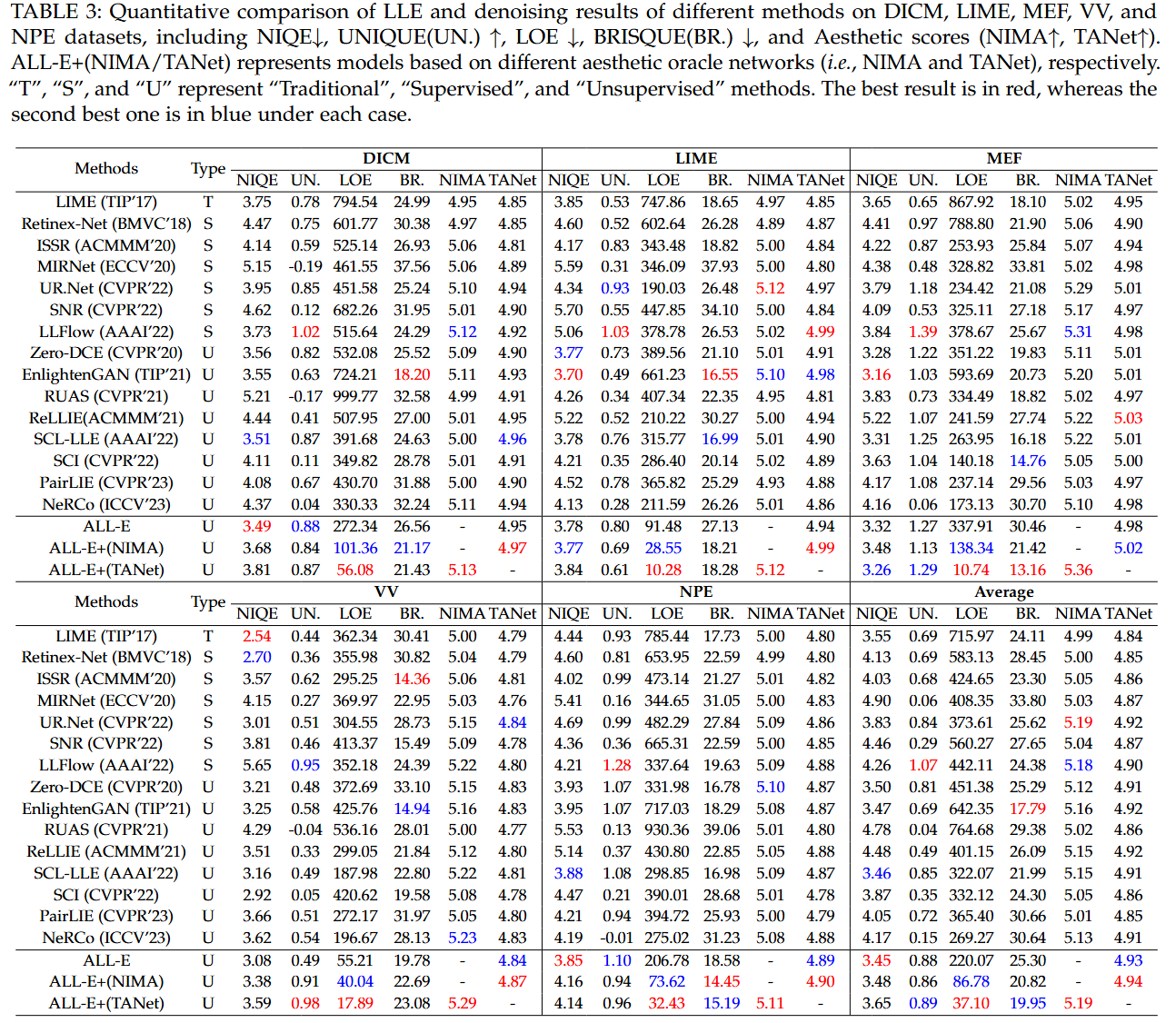
Visual quality comparison
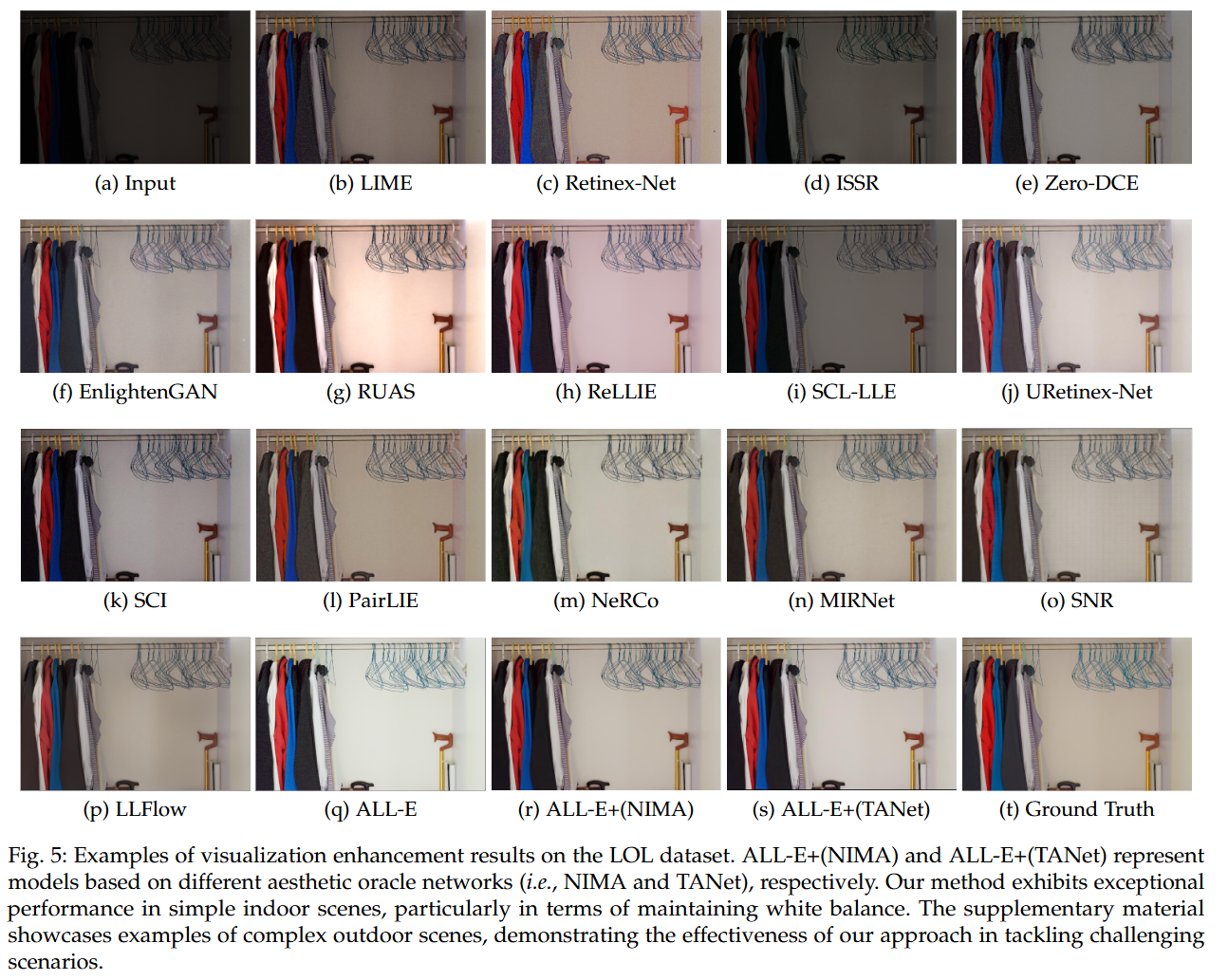
Human subjective survey
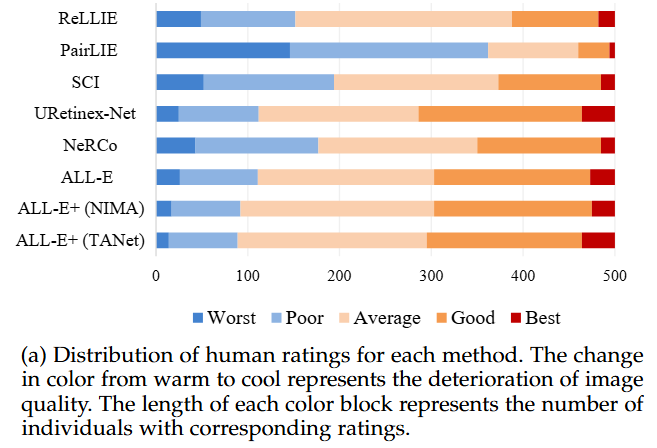
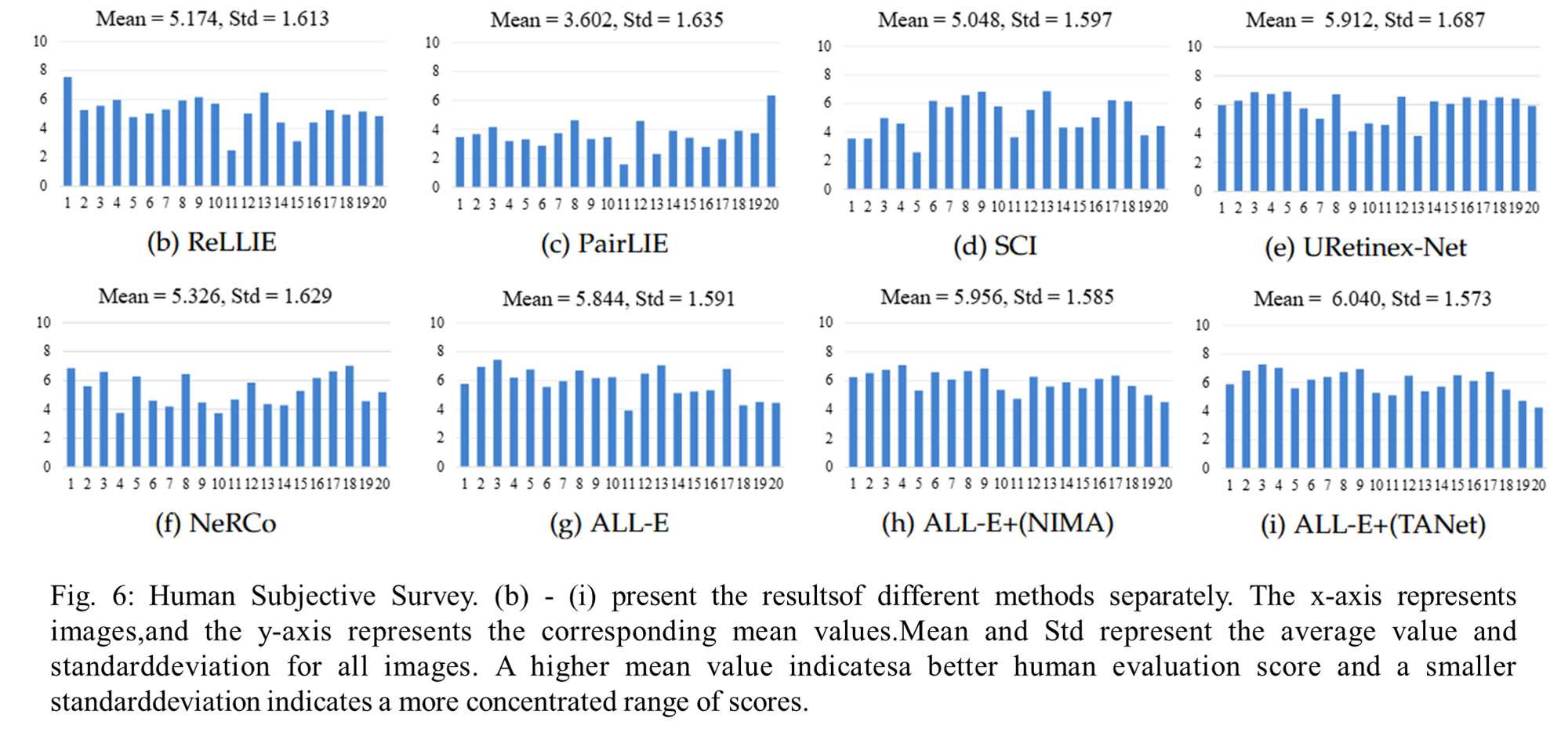
Experiments of Denoising
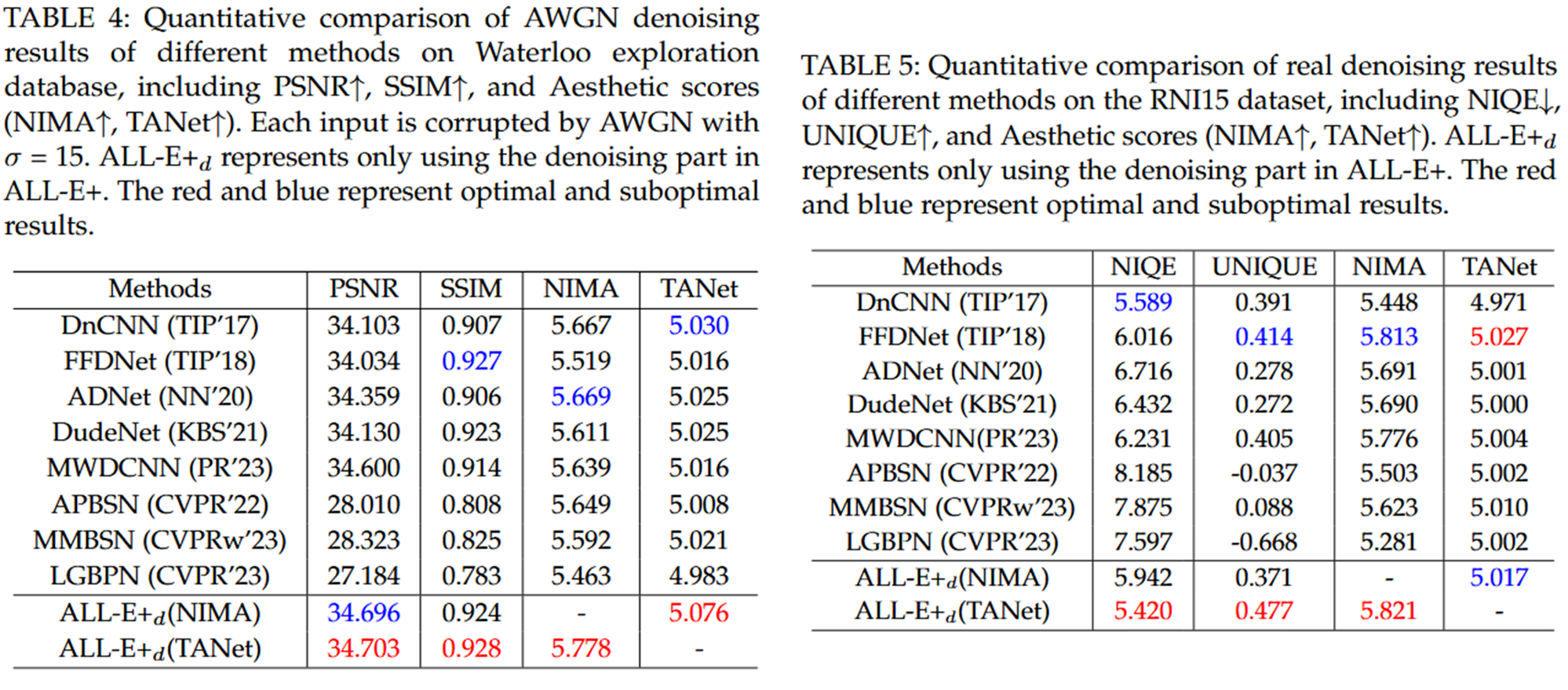
Ablation Studies
Contribution of Action Space Setting and Aesthetic Subjective Reward
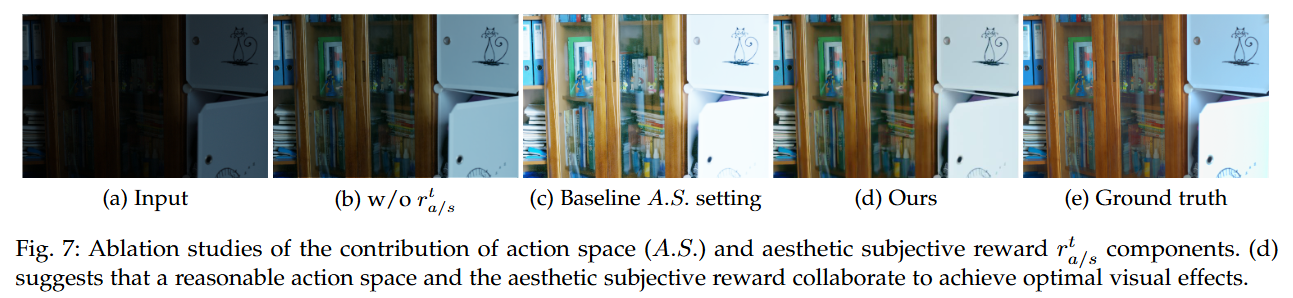
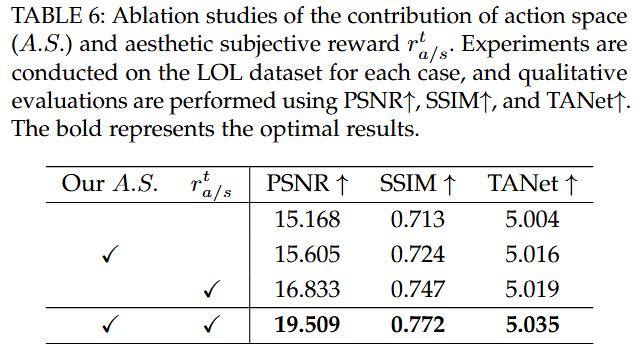
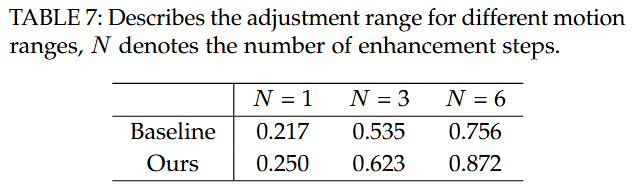
Contribution of Aesthetic Reward and Aesthetic Oracle Network
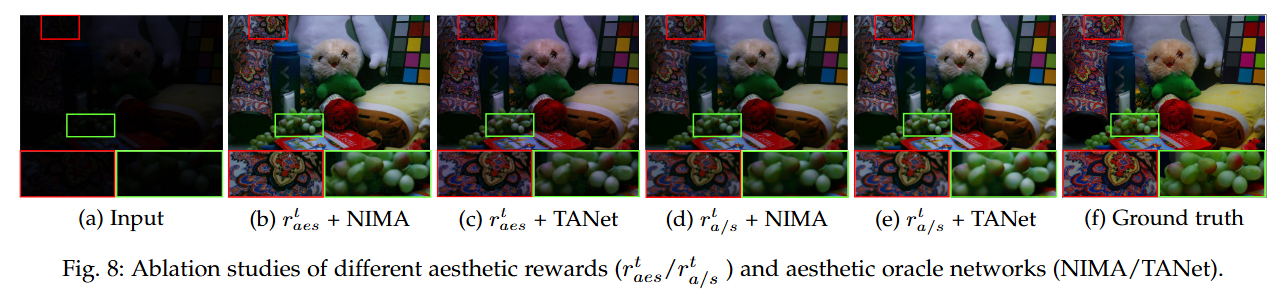
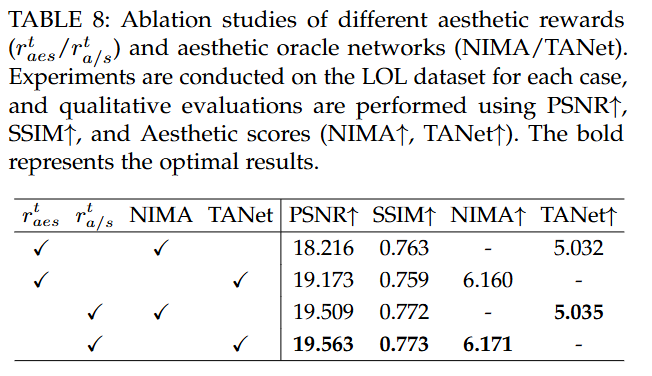
Aesthetics-guided Denoising Module
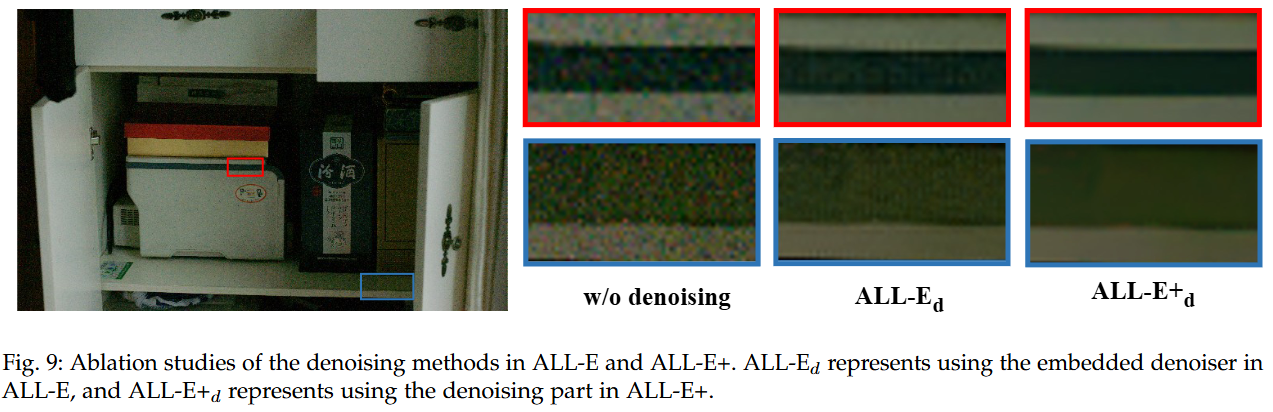
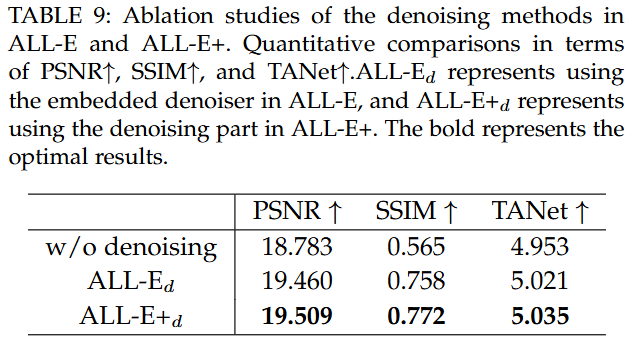
The Number of Enhancement Steps
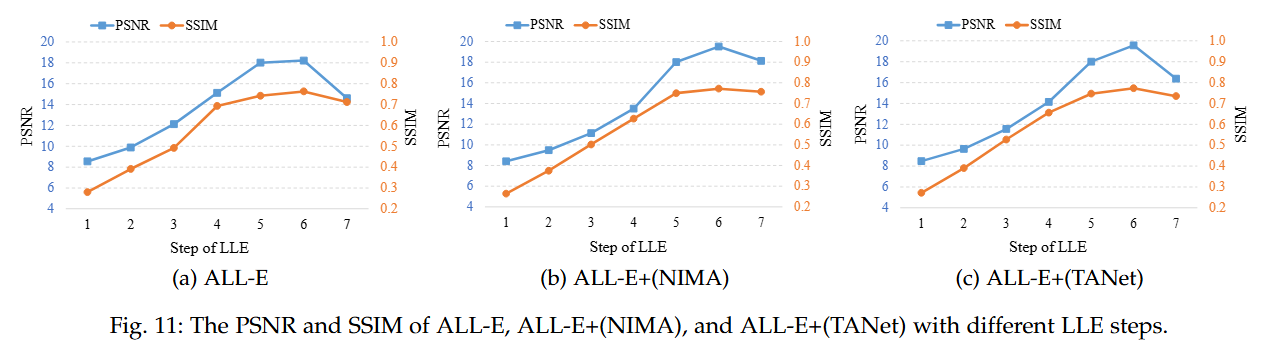
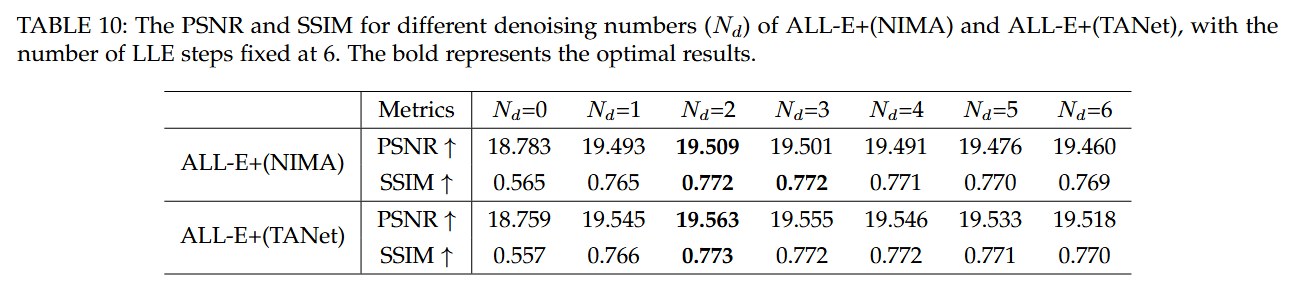
Plug and Play into other Supervised/Unsupervised LLE Networks
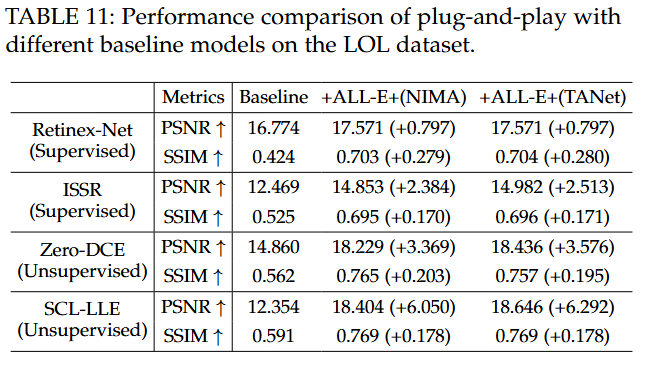
Materials
Homepage | PDF of ALL-E| Code of ALL-E| PDF of ALL-E+| Code of ALL-E+
Citation
ALL-E
@@inproceedings{li2023alle, title = {ALL-E: Aesthetics-guided Low-light Image Enhancement}, author ={Ling Li and Dong Liang and Yuanhang Gao and Sheng-Jun Huang and Songcan Chen}, booktitle = {Proceedings of the Thirty-Second International Joint Conference on Artificial Intelligence, {IJCAI-23}}, publisher = {International Joint Conferences on Artificial Intelligence Organization}, year={2023}, month = {8}, pages = {1062--1070} }
@ARTICLE{10938859, author={Liang, Dong and Gao, Yuanhang and Li, Ling and Xu, Zhengyan and Huang, Sheng-Jun and Chen, Songcan}, journal={IEEE Transactions on Pattern Analysis and Machine Intelligence}, title={Aesthetics-guided Low-light Enhancement}, year={2025}, volume={}, number={}, pages={1-18}, keywords={Noise reduction;Visualization;Noise;Image enhancement;Training;Reinforcement learning;Brightness;Image restoration;Image color analysis;Noise level;image aesthetics assessment;low-light enhancement;image denoising;reinforcement learning}, doi={10.1109/TPAMI.2025.3554639}} }
Contact
If you have any questions, please contact liangdong@nuaa.edu.cn.